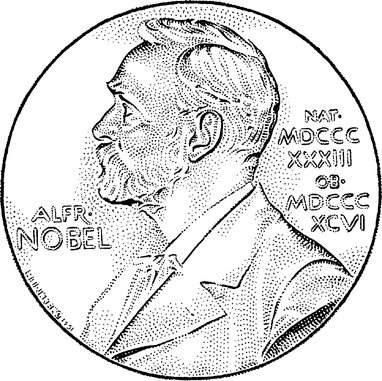
What would success look like, when it comes to the question of consciousness?
Of course it depends which of the many intersecting tribes who dispute or share the territory you belong to. Robot builders and AI designers have known since Turing that their goal is a machine whose responses cannot be distinguished from those of a human being. There’s a lot wrong with the Turing Test, but I still think it’s true that if we had a humanoid robot that could walk and talk and interact like a human being in a wide range of circumstances, most people wouldn’t question whether it was conscious or not. We’d like a theory to go with our robot, but the main thing is whether it works. Even if we knew it worked in ways that were totally unlike biological brains, it wouldn’t matter – planes don’t fly the birds do, but so what, it’s still flying. Of course we’re a million miles from such a perfectly human robot, but we sort of know where we’re going.
It’s a little harder for neurologists; they can’t rely quite so heavily on a practical demonstration, and reverse engineering consciousness is tough. Still, there are some feats that could be pulled off that would pretty much suggest the neurologists have got it. If we could reliably read off from some scanner the contents of anyone’s mind, and better yet, insert thoughts and images at will, it would be hard to deny that the veil of mystery had been drawn back quite a distance. It would have to be a general purpose scanner, though; one that worked straight away for all thoughts in any person’s brain. People have already demonstrated that they can record a pattern from one subject’s brain when that subject is thinking a known thought, and then, in the same session with the same subject, recognise that same pattern as a sign of the same thought. That is a much lesser achievement, and I’m not sure it gets you a cigar, let alone the Nobel prize.
What about the poor philosophers? They have no way to mount a practical demonstration, and in fact no such demonstration can save them from their difficulties. The perfectly human robot does not settle things for them; they tell it they can see it appears to be able to perform a range of ‘easy’ cognitive tasks, but whether it really knows anything at all about what it’s doing is another matter. They doubt whether it really has subjective experience, even though it assures them that it’s own introspective evidence says it does. The engineer sitting with them points out that some of the philosophers probably doubt whether he has subjective experience.
“Oh, we do,” they admit, “in fact many of us are pretty sure we don’t have it ourselves. But somehow that doesn’t seem to make it any easier to wrap things up.”
Nor are the philosophers silenced by the neurologists’ scanner, which reveals that an apparently comatose patient is in fact fully aware and thinking of Christmas. The neurologists wake up the subject, who readily confirms that their report is exactly correct. But how do they know, ask the philosophers; you could be recording an analogue of experience which gets tipped into memory only at the point of waking, or your scanner could be conditioning memory directly without any actual experience. The subject could be having zomboid dreams, which convey neural data, but no actual experience.
“No, they really couldn’t,” protest the neurologists, but in vain.
So where do philosophers look for satisfaction? Of course, the best thing of all is to know the correct answer. But you can only believe that you know. If knowledge requires you to know that you know, you’re plummeting into an infinite regress; if knowing requires appropriate justification then you’re into a worm-can opening session about justification of which there is no end. Anyway, even the most self-sufficient of us would like others to agree, if not recognise the brilliance of our solution.
Unfortunately you cannot make people agree with you about philosophy. Physicists can set off a bomb to end the argument about whether e really equals mc squared; the best philosophers can do is derive melancholy satisfaction from the belief that in fifty years someone will probably be quoting their arguments as common sense, though they will not remember who invented them, or that anyone did. Some people will happen to agree with you already of course, which is nice, but your arguments will convert no-one; not only can you not get people to accept your case; you probably can’t even get them to read your paper. I sympathised recently with a tweet from Keith Frankish lamenting how he has to endlessly revisit bits of argument against his theory of illusionism, one’s he’s dealt with many times before (oh, but illusions require consciousness; oh, if it’s an illusion, who’s being deceived…). That must indeed be frustrating, but to be honest it’s probably worse than that; how many people, having had the counter-arguments laid out yet again, accept them or remember them accurately? The task resembles that of Sisyphus, whose punishment in Hades was to roll a boulder up a hill it invariably rolled down again. Camus told us we must imagine Sisyphus happy, but that itself is a mental task which I find undoes itself every time I stop concentrating…
I suppose you could say that if you have to bring out your counter-arguments regularly, that itself is some indicator of having achieved some recognition. Let’s be honest, attention is what everyone wants; moral philosophers all want a mention on The Good Place, and I suppose philosophers of mind would all want to be namechecked on Westworld if Julian Jaynes hadn’t unaccountably got that one sewn up.
Since no-one is going to agree with you, except that sterling band who reached similar conclusions independently, perhaps the best thing is to get your name associated with a colourful thought experiment that lots of people want to refute. Perhaps that’s why the subject of consciousness is so full of them, from the Chinese Room to Mary the Colour Scientist, and so on. Your name gets repeated and cited that way, although there is a slight danger that it ends up being connected forever with a point of view you have since moved on from, as I believe is the case with Frank Jackson himself, who no longer endorses the knowledge argument exemplified by the Mary story.
Honestly, though, being the author of a widely contested idea is second best to being the author of a universally accepted one. There’s a Borges story about a deposed prince thrown into a cell where all he can see is a caged jaguar. Gradually he realises that the secrets of the cosmos are encoded in the jaguar’s spots, which he learns to read; eventually he knows the words of magic which would cast down his rival’s palace and restore him to power; but in learning these secrets he has attained enlightenment and no longer cares about earthly matters. I bet every philosopher who reads this story feels a mild regret; yes, of course enlightenment is great, but if only my insights allowed me to throw down a couple of palaces? That bomb thing really kicked serious ass for the physicists; if I could make something go bang, I can’t help feeling people would be a little more attentive to my corpus of work on synthetic neo-dualism…
Actually, the philosophers are not the most hopeless tribe; arguably the novelists are also engaged in a long investigation of consciousness; but those people love the mystery and don’t even pretend to want a solution. I think they really enjoy making things more complicated and even see a kind of liberation in the indefinite exploration; what can you say for people like that!