Could Artificial Intelligence be the tool that finally allows us understand the natural kind?
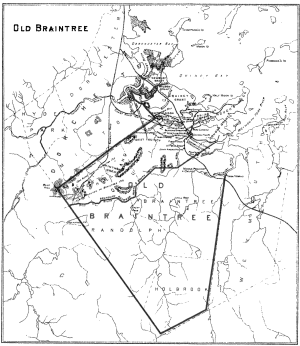
We’ve talked before about the possibility; this Nautilus piece explains that scientists at the Max Planck Institute Of Neurobiology have come up with a way of using ‘neural’ networks to map, well, neural networks. There has been a continued improvement in our ability to generate images of neurons and their connections, but using those abilities to put together a complete map is a formidable task; the brain has often been described as the most complex object in the universe and drawing up a full schematic of its connections is potentially enough work for a lifetime. Yet that map may well be crucial; recently the idea of a ‘connectome’ roughly equivalent to the ‘genome’ has become a popular concept, one that suggests such a map may be an essential part of understanding the brain and consciousness. The Max Planck scientists have developed an AI, called ‘SyConn’ which tu4ns images into maps automatically with very high accuracy. In principle I suppose this means we could have a complete human connectome in the not-too-distant future.
How much good would it do us, though? It can’t be bad to have a complete map, but there are three big problems. The first is that we can already be pretty sure that connections between neurons are not the whole story. Neurons come in many different varieties, ones that pretty clearly seem to have different functions – but it’s not really clear what they are. They operate with a vast repertoire of neurotransmitters, and are themselves pretty complex entities that may have genuine computational properties all on their own. They are supported by a population of other, non-networked cells that may have a crucial role in how the overall system works. They seem to influence each other in ways that do not require direct connection; through electromagnetic fields, or even through DNA messages. Some believe that consciousness is not entirely a matter of network computation anyway, but resides in quantum or electrical fields; certainly the artificial networks that were originally inspired by biological neurology seem to behave in ways that are useful but quite distinct from those of human cognition. Benjamin Libet thought that if only he could do the experiment, he might be able to demonstrate that a sliver of the brain cut out from its neighbouring tissue but left in situ would continue to do its job. That, surely, is going too far; the brain didn’t grow all those connections with such care for nothing. The connectome may not be the full story, but it has to be important.
The second problem, though, is that we might be going at the problem from the wrong end. A map of the national road network tells us some useful things about trade, but not what it is or, in the deeper sense, how it works. Without those roads, trade would not occur in anything like the same form; blockages and poor connections may hamper or distort it, and in regions isolated by catastrophic, um, road lesions, trade may cease altogether. Of course to understand things properly we should need to know that there are different kinds of vehicle doing different jobs on those roads, that places may be connected by canals and railways as well as roads, and so on. But more fundamentally, if we start with the map, we have no idea what trade really is. It is, in fact, primarily driven and determined by what people want, need, and believe, and if we fall into the trap of thinking that it is wholly determined by the availability of trucks, goods, and roads we shall make a serious mistake.
Third, and perhaps it’s the same problem in different clothes, we still don’t have any clear and accepted idea of how neurology gives rise to consciousness anyway. We’re not anywhere near being able to look at a network and say, yup, that is (or could be) conscious, if indeed it is ever possible to reach such a conclusion.
So do we really even want a map of the connectome? Oh yes…